The New Reality of Scientific Research in 2025
The scientific community is facing an unprecedented challenge in 2025. Sweeping research funding cuts across major institutions are sending shockwaves through laboratories nationwide.
For many, the outlook is grim. In an interview with NPR, Dr. Jo Handelsman (former White House Associate Director for Science and current director of the Wisconsin Institute for Discovery) said that these cuts "will destroy science in the United States" and "break our universities, our medical centers and the entire engine for scientific discovery."
The consequences are already visible:
- Johns Hopkins eliminating over 2,200 staff positions
- Stanford announcing a hiring freeze
- Promising graduate students receiving rejection letters citing "government decisions"
In this challenging environment, AI for enhancing research productivity during funding constraints has emerged as a critical strategy for research teams facing difficult budgetary decisions.
This article provides a few practical, AI-powered approaches to maintain research momentum despite reduced resources, helping your team do more with less without compromising scientific integrity.
Understanding the 2025 Funding Crisis in Context
Scientific funding has always experienced cycles, but the 2025 cuts represent a particularly sharp deviation from the post-WWII compact. For nearly 70 years, the United States has carried out its national research agenda primarily through university-based research rather than building dedicated government research facilities.
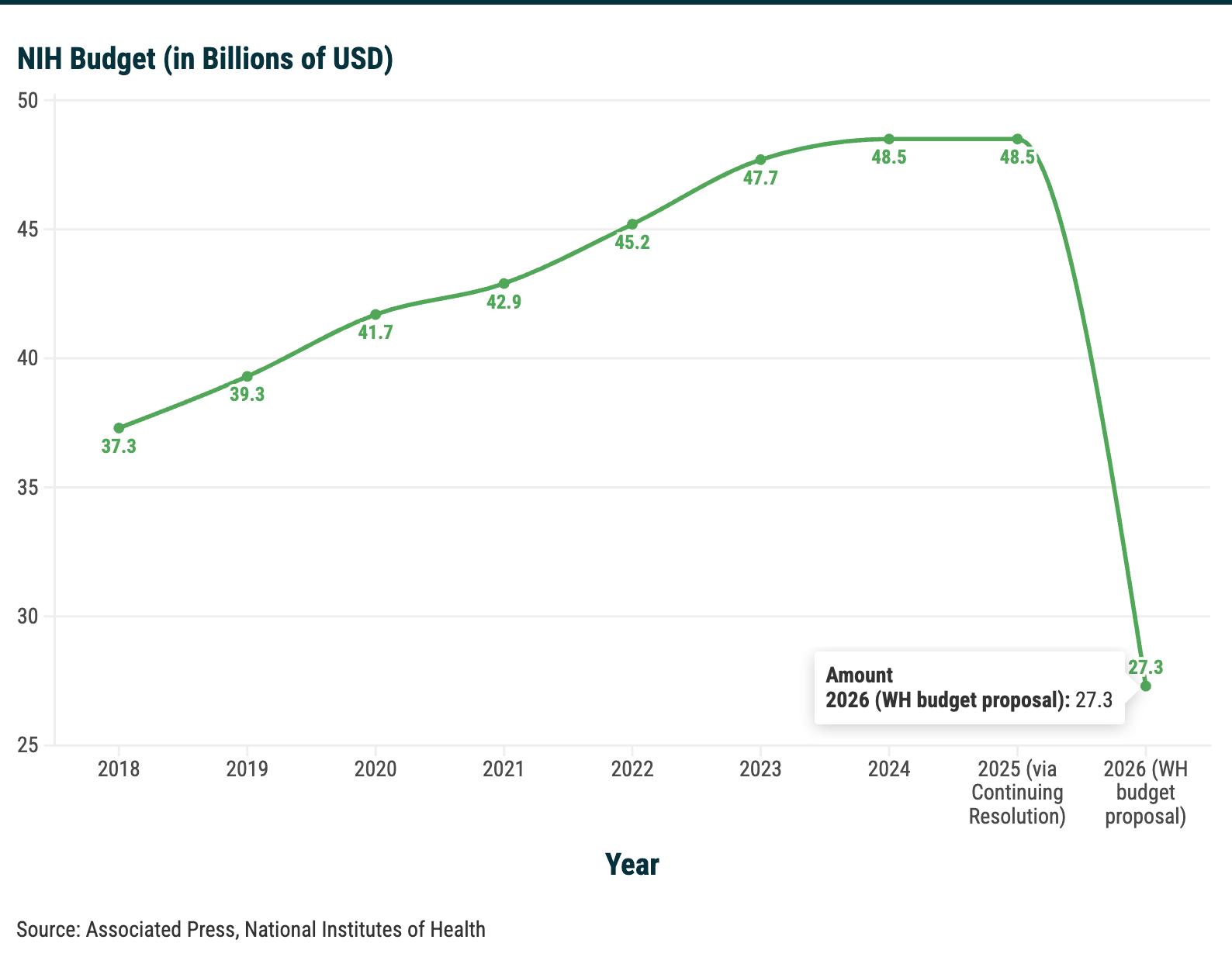
Current cuts disproportionately affect biomedical research, with potential ripple effects threatening key research targets such as vaccine development and cancer treatments.
AI as a Solution: Amplifying Research Capacity
AI presents a powerful counterbalance to funding limitations. By automating routine tasks and augmenting human capabilities, AI tools enable research teams to maintain, and sometimes even increase, their scientific output despite reduced budgets.
Some of the strategic advantages offered by AI for resource-constrained labs include:
- Automate time-intensive workflows: Modern AI systems can conduct preliminary literature reviews in hours instead of weeks, organize citations according to relevance hierarchies, and automatically flag papers with methodological similarities to your research questions.
- Transform information processing: Natural language models now excel at synthesizing information across hundreds of papers, generating comprehensive research summaries, and identifying emerging patterns that might suggest promising hypotheses human researchers could otherwise miss.
- Streamline grant acquisition: From competitive landscape analysis to formatting requirements, AI assistants can support researchers throughout the grant development process, helping teams submit more polished and compelling proposals despite reduced administrative support.
Direct, measurable benefits of implementing AI tools include:
- Significant reduction in time spent on administrative tasks
- AI-powered analysis can identify patterns and connections across datasets that might take humans months to recognize
- Improved quality control through automated validation of methodologies
Maximizing Literature Review Efficiency with AI
One of the most time-consuming aspects of research is the comprehensive literature review. Traditional approaches require weeks or months of meticulous searching, reading, and synthesizing. This time is now a luxury that resource-constrained labs can no longer afford.
AI tools for academic paper organization can dramatically reduce this burden while maintaining quality.
Practical benefits include:
- Rapid identification of methodology gaps in existing literature
- Automatic flagging of contradictory findings across studies
- Visualization of research trends that illuminate promising directions
- Significant time savings that can be redirected to experimental work
Read our in-depth guide to using AI for literature review (internal link to be posted later).
Optimizing Resource Allocation Through AI Validation
Traditional, unassisted, trial-and-error approaches to experimentation have become more unsustainable due to resource bottlenecks. AI-powered research validation tools can help researchers and institutions do more with less through preventing costly dead-ends by:
- Predicting experimental outcomes based on existing literature and datasets
- Identifying optimal parameters for initial experiments
- Suggesting the most efficient sequence of experiments
- Flagging potential methodological weaknesses before resources are committed
MIT's Materials Science and Engineering department demonstrates this approach with CRESt, an AI-powered assistant that helps researchers control scientific experiments through voice commands. The system "uses a trained algorithm to select the next set of experimental parameters to evaluate," effectively guiding researchers toward the most promising pathways.
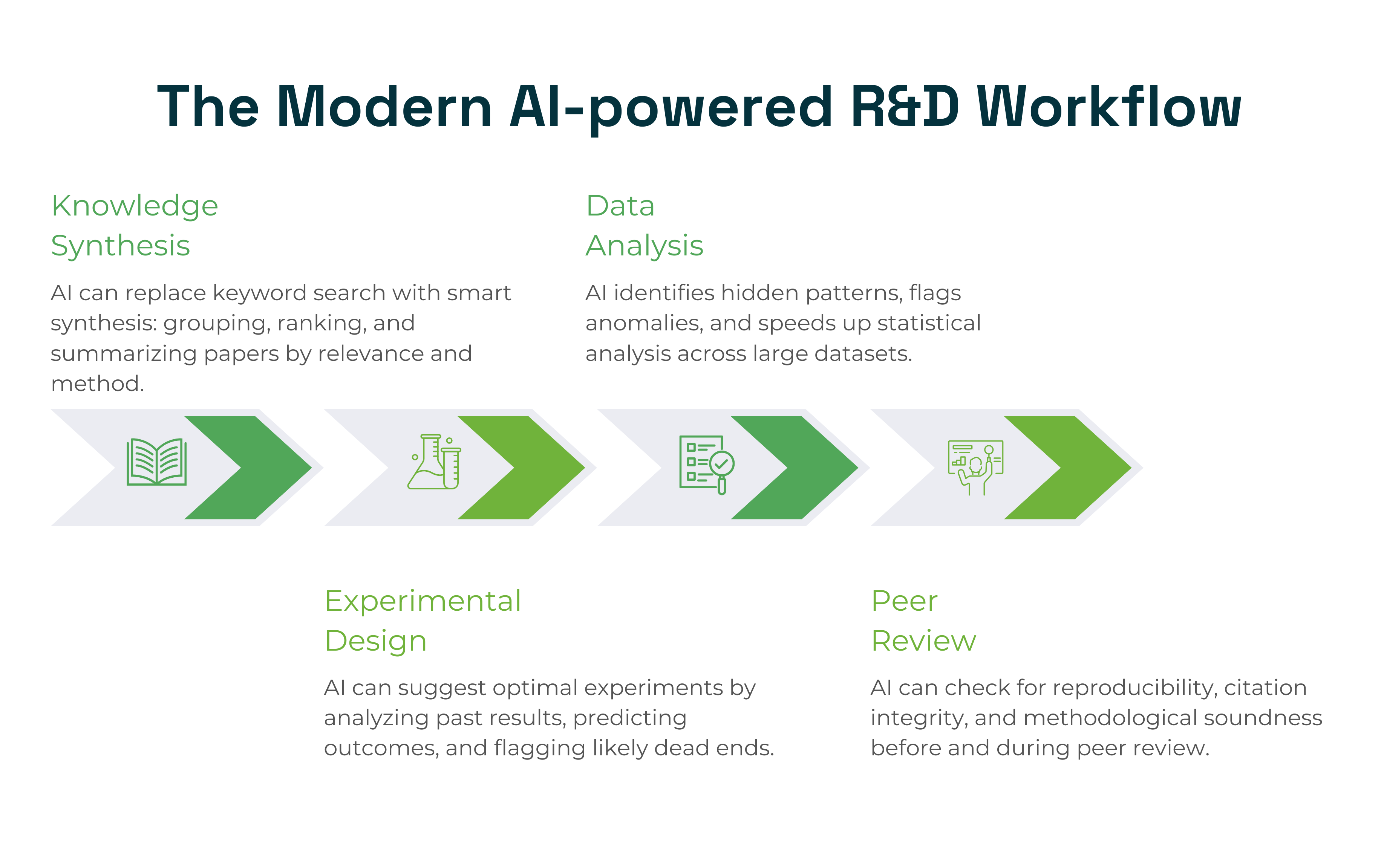
Practical Steps for Implementation
Implementing AI tools for research productivity doesn't require enormous up-front investments. Here's a practical approach for resource-constrained labs:
- Start with free or low-cost options: Many powerful AI research tools offer free tiers or academic pricing. Begin with these to demonstrate value before committing resources.
- Target high-impact processes first: Identify your lab's most resource-intensive processes (literature reviews, experimental design, data analysis) and focus AI implementation on these areas for maximum return.
- Build internal expertise gradually: Designate a team member to develop expertise with each new tool rather than attempting to train everyone simultaneously.
- Measure concrete outcomes: Track specific metrics like time saved, experimental success rates, and publication outputs to quantify the return on investment.
- Maintain scientific oversight: Remember that AI tools augment rather than replace scientific judgment. The most successful implementations maintain a balance between AI insights and researcher expertise.
Looking Forward: Adaptation as Opportunity
While the 2025 funding crisis presents significant challenges, it may ultimately accelerate beneficial transformations in research methodologies. Labs that successfully integrate AI tools now can weather current constraints and potentially emerge stronger when funding environments eventually improve.
As research institutions adapt to these new realities, those who embrace AI-enhanced workflows may discover unexpected benefits: more efficient processes, broader collaboration networks, and research directions that might otherwise have remained unexplored.
For researchers navigating these challenging times, AI tools offer not just a stopgap measure but potentially a new paradigm for conducting more efficient, collaborative, and productive science.